Harnessing the Power of Business in Machine Learning: A Comprehensive Guide
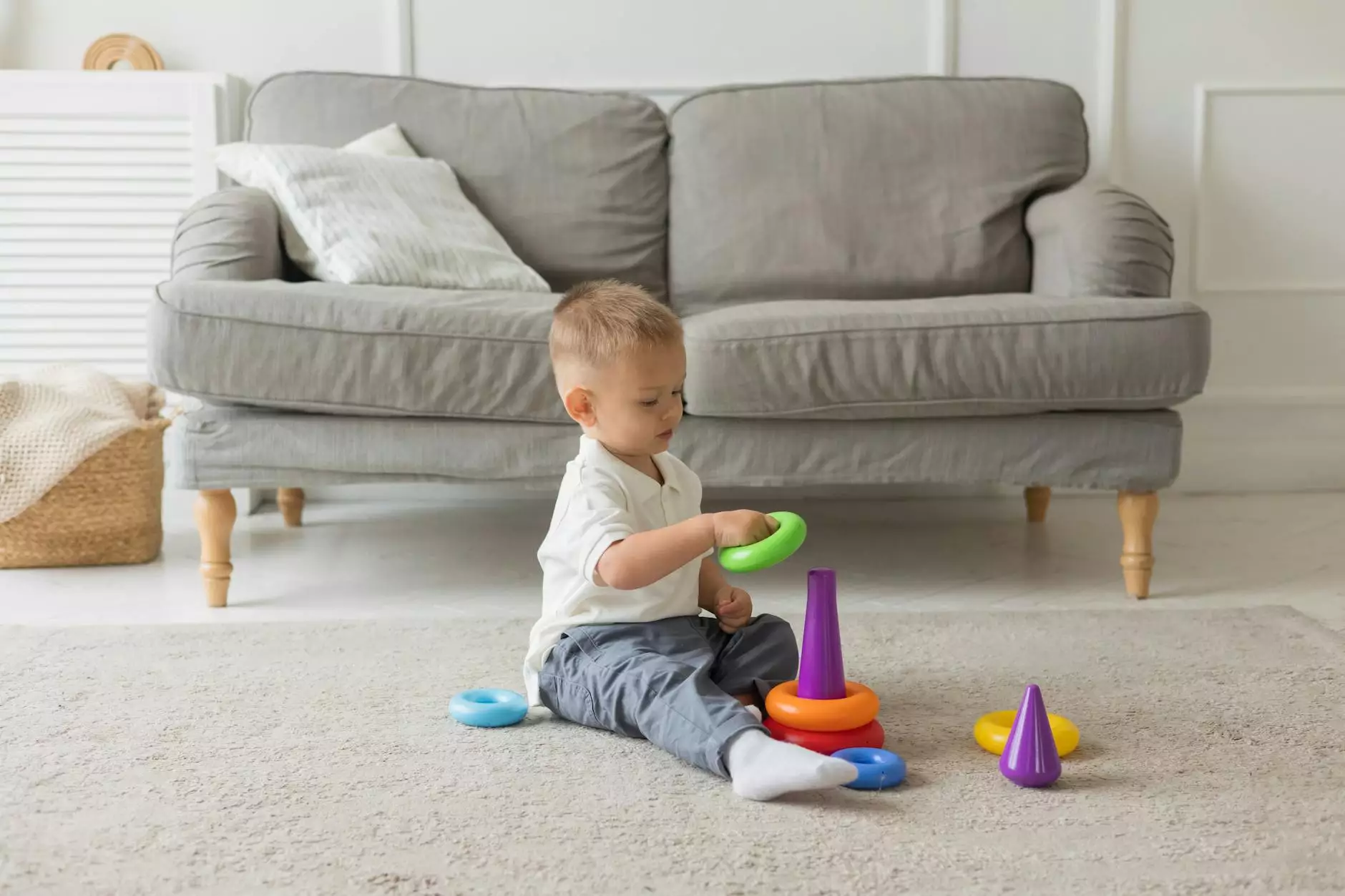
In today's technological era, business and machine learning (ML) are deeply intertwined, creating unprecedented opportunities for innovation and efficiency. The rise of machine learning consulting has paved the way for organizations to leverage data effectively, enabling businesses to unlock insights that drive growth. This article will delve into the multifaceted relationship between business and machine learning, exploring its implications across various sectors and offering strategies to harness ML effectively for your business success.
Understanding Machine Learning and Its Business Impact
Machine learning refers to a subset of artificial intelligence (AI) that empowers systems to learn from data, identify patterns, and make decisions with minimal human intervention. Businesses across various industries are beginning to recognize the potent benefits of integrating ML into their operations, which can lead to:
- Improved Efficiency: ML algorithms can automate routine tasks, allowing organizations to allocate resources more effectively.
- Enhanced Decision-Making: With predictive analytics, businesses can make data-driven decisions that reduce uncertainty.
- Personalized Customer Experiences: By analyzing customer data, businesses can tailor products and services to meet specific consumer needs.
- Cost Reduction: Identifying inefficiencies through data analysis can significantly lower operational costs.
The Role of Data in Machine Learning for Business
At the core of machine learning lies data—a critical asset for any organization. Data serves as the foundation for training ML models, which generate valuable insights. However, not all data is created equal; the quality and relevance of the data significantly influence the performance of ML models. Here are some considerations for effective data management:
1. Data Collection
Businesses must implement effective strategies for data collection to ensure they capture relevant information. This can involve:
- Surveys and feedback forms
- Monitoring online interactions and behaviors
- Utilizing third-party data sources
2. Data Quality
Ensuring high-quality data is essential. Businesses should focus on:
- Data cleaning to remove inaccuracies and duplicates
- Regular audits to maintain data integrity
3. Data Security
With increasing regulations around data privacy, it is important for businesses to implement robust security measures. This includes:
- Encryption of sensitive data
- Compliance with regulations like GDPR and CCPA
Applications of Machine Learning in Business
The applications of machine learning in business are vast and transformative. Here are several key areas where businesses can implement machine learning solutions:
1. Marketing
In marketing, machine learning can enhance customer segmentation, targeting, and campaign effectiveness. Businesses can analyze consumer behavior patterns to create personalized marketing strategies that resonate with their audience.
2. Financial Services
Machine learning is revolutionizing the finance sector by improving risk assessment, fraud detection, and algorithmic trading. Financial institutions can quickly analyze vast amounts of transaction data to identify potential fraud and make real-time financial decisions.
3. Supply Chain Management
By predicting demand and optimizing inventory levels, businesses can streamline their supply chain processes. Machine learning algorithms can forecast fluctuations in demand based on historical data, seasonality, and market trends.
4. Human Resources
In HR, machine learning can facilitate talent acquisition and employee engagement. Recruitment tools utilize ML to streamline the hiring process by matching candidates' skills with job requirements effectively.
Challenges of Implementing Machine Learning in Business
While the potential benefits of machine learning for businesses are substantial, there are challenges that organizations may face during implementation:
1. Lack of Expertise
Many businesses lack the technical expertise necessary to develop and manage machine learning systems. Hiring qualified data scientists and ML engineers can be a significant investment, which may deter some organizations from embracing ML.
2. Integration with Existing Systems
Integrating machine learning solutions with legacy systems can present technical difficulties. Organizations must consider compatibility and ensure that their infrastructure supports new technologies.
3. Ethical Considerations
The rise of machine learning and AI has raised ethical concerns surrounding bias, transparency, and job displacement. Businesses must approach ML implementation thoughtfully, weighing the potential impacts on stakeholders and society.
Best Practices for Leveraging Machine Learning in Your Business
To successfully leverage machine learning, organizations should consider the following best practices:
1. Start Small
Businesses should begin by implementing small-scale ML projects that address specific problems. This helps in gauging the effectiveness of machine learning solutions before scaling up.
2. Foster a Culture of Data-Driven Decision Making
Encourage employees at all levels to rely on data when making decisions. Providing training on data interpretation and machine learning can enhance employees' understanding and engagement with these technologies.
3. Collaborate with Experts
Engaging with machine learning consultants can provide valuable expertise in designing and implementing ML strategies tailored to your business needs.
4. Continuously Monitor and Optimize
Data and markets evolve; businesses must regularly assess and optimize their ML models to ensure they remain accurate and effective.
Future Trends in Machine Learning and Business
Looking ahead, some key trends in machine learning and business will likely emerge:
1. Increased Automation
Further advancements in machine learning will lead to greater automation of routine and complex tasks across industries, enhancing productivity.
2. Enhanced AI Ethics
As public awareness of ethical considerations grows, businesses will need to prioritize transparency and fairness in their machine learning applications.
3. Collaborative AI
Organizations will increasingly adopt collaborative systems where humans and machines work together, leveraging the strengths of both.
Conclusion
In summary, the intersection of business and machine learning presents significant opportunities for growth, efficiency, and innovation. By understanding the role of data, exploring practical applications, and overcoming challenges, organizations can revolutionize their operations and stay competitive in the digital landscape. As machines learn, the businesses that adapt will thrive, leading us into an era where intelligent decision-making is paramount.
Embrace the future of machine learning and position your business at the forefront of this technological advancement. Discover how machine learning consulting can help your organization navigate this landscape effectively, ensuring sustained success and growth.
ml blog